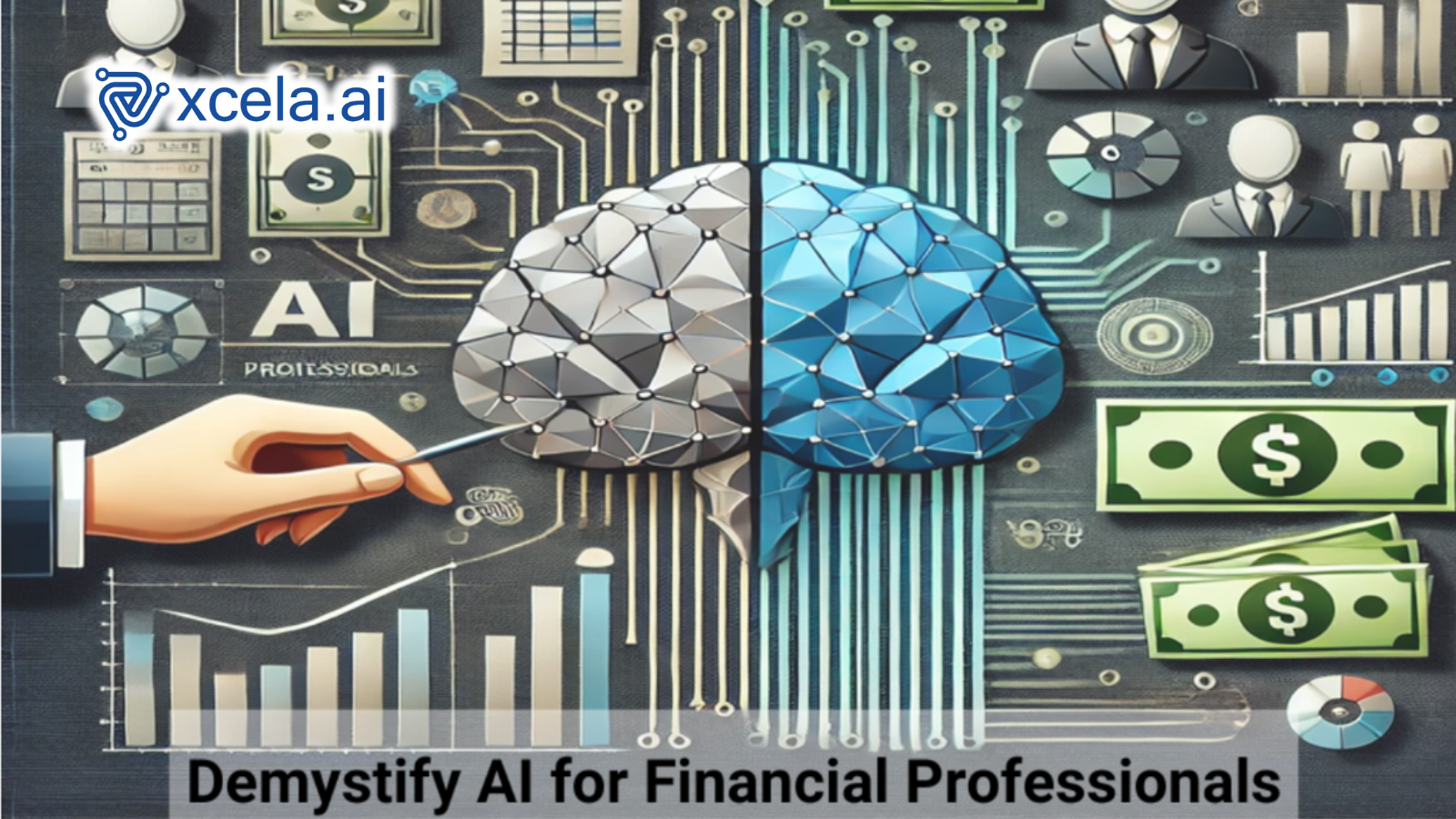
Demystifying AI for Financial Professionals
Introduction
AI is everywhere today, and for financial professionals, it’s no longer just a trend—it’s a necessity. From automating routine tasks to providing personalized client solutions, AI is transforming the way businesses function.
In Part 1, we explored how AI is reshaping life insurance sales. Now, in Part 2, we’ll dive deeper into what AI really is and how it can impact your business.
But before we get into that, let’s look at some fascinating facts about AI.
6 Facts About AI
- AI is all around us: Nearly 77% of today’s devices — from smartphones to home assistants—use AI in some form.
- AI skills are in high demand: Since 2013, jobs requiring AI expertise have increased by 4.5 times, reflecting its increasing importance in the workforce.
- The AI market is booming: The AI industry is expected to skyrocket from $214.6 billion in 2024 to an astonishing $1.3 trillion by 2030.
- AI will create more jobs than it replaces: While AI may displace 85 million jobs, it’s also expected to create 97 million new roles in various industries.
- AI’s economic impact is massive: By 2030, AI could add $15.7 trillion to the global economy, making it a huge driver of economic growth.
- AI assistants will outnumber humans: By 2024, there will be 8.4 billion AI-powered assistants worldwide, outnumbering people on the planet.
PBJ (Practical, Balanced, Just-right AI) – Xcela’s Approach
- Practical: AI that’s easy to use and applies directly to real-world scenarios—no overcomplicated processes or unnecessary steps, just like a simple, satisfying peanut butter and jelly sandwich.
- Balanced: AI that strikes the perfect balance between automation and human input, ensuring both efficiency and accuracy without sacrificing quality and the human interactions
- Just-right: AI that’s tailored to your needs, delivering results that are neither too complex nor too basic—just like a well-made PBJ, it’s exactly what you need, when you need it.
Just like a PBJ sandwich is a classic, no-fuss solution for hunger, PBJ AI is the practical, balanced, and just-right approach to implementing artificial intelligence in your business. It’s easy to understand, works seamlessly, and delivers results without overcomplicating things.
What Do All the AI Acronyms and Terms Mean?
Here are some key AI acronyms and terms that financial professionals should be familiar with before diving deeper into the world of AI:
- GenAI (Generative AI): AI that creates new content—whether it’s text, images, or videos—based on the data it’s trained on. Think of it as the technology behind AI writing an email or creating a piece of artwork.
- LLM (Large Language Model): An AI model trained on massive amounts of text to understand and generate human-like language. It’s essentially teaching a machine how to read and write at a highly advanced level.
- Multi-modal LLM: A more advanced version of LLM that can process multiple types of data, like text, images, and videos, all at once.
- AI (Artificial Intelligence): Machines that mimic human intelligence, assisting with tasks like learning, problem-solving, and understanding language.
- RAG (Retrieval-Augmented Generation): This technique helps AI provide better answers by retrieving information from external knowledge sources, like databases or documents before generating responses.
- RAFT (Reliable, Accountable, Fair, Transparent): A methodology to ensure the responsible and ethical use of AI. It enhances LLMs, helping them retrieve the most relevant information and improving their accuracy and transparency when generating responses. More on this in our next article.
- Machine Learning (ML): A way for computers to learn from data and improve their performance over time.
- Feedback Learning (FL): AI learns from human feedback to improve its outputs.
- Human-in-the-Loop (HITL): An AI workflow where humans will be stepping in to guide or correct the machine when necessary.
What is AI and Machine Learning?
Now that we’ve covered some key acronyms and terms, let’s break down what AI and machine learning really mean—in the simplest way possible.
Artificial Intelligence (AI) is the technology that allows machines to think and act like humans. It enables computers to solve problems, recognize patterns, and make decisions—similar to how we do. AI is behind everything from voice assistants to personalized recommendations, and even chatbots.
Machine Learning (ML) is a specific type of AI that enables computers to learn from data. Imagine teaching a child to recognize animals by showing them pictures of cats and dogs. In the same way, ML trains computers to make decisions by processing large amounts of data. The more data it analyzes, the better it gets at identifying patterns and predicting outcomes.
In short, AI and ML are powerful tools that help automate complex tasks, save time, and boost efficiency, helping your business run smarter and faster.
What is Building Your LLM vs Fine-Tuning An LLM?
When integrating AI into your business, you might ask whether it’s better to build your own LLM (Large Language Model) from scratch or fine-tune an existing one.
Building your LLM means starting from the ground up by training a model on massive amounts of data. This process requires significant time, financial investment, and technical expertise. It’s complex but can result in a highly customized model tailored to very specific needs.
Fine-tuning an LLM, on the other hand, involves taking an already trained model and adapting it to your specific requirements by training it on more targeted data. This approach is faster, more affordable, and efficient, as the model already has a foundational understanding of language patterns.
For most businesses, fine-tuning is the ideal solution, offering customization for specialized tasks without the hefty investment needed to build an LLM from scratch.
Open Source vs. Closed Source LLMs
When deciding between Open Source and Closed Source LLMs, it’s important to understand the differences and what they mean for your business.
Open Source LLMs – like Mistral, Falcon, Llama models – are freely accessible for anyone. You can use, modify, and even fine-tune these models to meet your specific needs. The datasets used to train these models are often available as well, offering flexibility and cost-effectiveness. Open source models are a great option if you want full control over your LLM and the freedom to customize it without paying high fees.
On the other hand, Closed Source LLMs, like OpenAI, are proprietary, very powerful and controlled by private companies, and the dataset used to train the model is not publicly available.
Another factor to consider is the number of parameters in an LLM, which refers to the size and complexity of the model. For example, a model with 7B parameters will be less powerful but cheaper to train than one with 40B parameters. Below, you can see that training a 7B LLM on A100 GPU from scratch could cost around $300K – while training a 40B LLM could run up to $1.74M.
You can use this LLM training cost calculator to estimate exact costs based on your needs.
Ultimately, the decision between open source and closed source depends on your business needs—whether you prioritize flexibility and cost or prefer the enhanced security and features of proprietary models.
Why Are PII, PCI, and PHI Important in LLMs?
When working with LLMs, it’s understanding the importance of PII (Personally Identifiable Information), PCI (Payment Card Information), and PHI (Protected Health Information) is crucial because these types of data are highly sensitive and must be protected to ensure privacy.
If an LLM processes this data without proper security protocols, it could lead to data breaches, identity theft, or violations of privacy laws. Mishandling PII, PCI, or PHI can also result in legal and financial penalties, especially in industries like healthcare and finance where data protection is heavily regulated.
Ensuring your AI system is compliant with regulations like GDPR and HIPAA is essential to keeping your clients’ information safe and maintaining trust.
BUILD v. BUY v. BLEND: Do You Need to Build Your Own AI LLM Solution?
When considering AI for your business, the question often arises: should you build, buy, or blend your AI solution?
Building your own LLM in-house can be appealing because it offers complete control and customization. However, this comes with significant costs and technical challenges. Training a large model from scratch can easily cost hundreds of thousands of dollars, and the expertise required to manage, fine-tune, and maintain it makes this option impractical for most businesses.
Additionally, ongoing feature tuning—the process of optimizing the model’s performance for specific tasks—requires deep technical knowledge and can be time-consuming.
On the other hand, buying a proprietary solution might seem simpler and faster, but it often lacks the flexibility and customization that many businesses need. It can also become expensive over time due to subscription costs and limited adaptability.
That’s where blending comes in—a combination of RAG, Open Source LLMs, and secure infrastructure provides a balanced approach. Blending allows businesses to leverage powerful AI models and customize features without the overwhelming cost and complexity of building from scratch. Feature tuning can still be applied to an existing model to optimize it for your business’s unique needs (if you have the experts in-house).
Still, challenges such as cost, expertise, and the upfront investment remain. In the next article of this 5-part series on AI for Life Insurance, we’ll dive deeper into the unique challenges of applying AI to selling life insurance and what to keep in mind as you move forward.
Looking to adopt AI without the technical burden? Contact Xcela today to explore how we can help grow your insurance business with customized AI solutions.
Originally posted by: Xcela